Adversarial Cases for Autonomous Vehicles
We witnessed unprecedented technological growth in the automotive industry in autonomous vehicles (AVs). It is driven by the intense efforts of many leading IT companies and hundreds of millions of kilometers of data acquired. However, despite the considerable effectiveness of Deep Learning (DL) algorithms in learning from data, they lack robustness to rare and adversarial cases.
If not recognized and adequately addressed by AVs, such cases may lead to accidents and fatalities. However, it is not enough to manually examine the few most apparent critical cases – a methodological and well-structured approach is needed. Due to its characteristics, real-life experiments cannot address the detection of adversarial issues, nor can they be extracted from the acquired data. Therefore, it is critical to take advantage of realistic simulation frameworks such as CARLA.
The ARCANE project objective is to develop a dedicated high-level application (plugin) capable of injecting adversarial cases into the simulated world in a methodological way. It should also collect the results of the AV response to be able to retrain it afterward and make it resistant to such cases in the future. This project proposes to address the search for adversarial cases by framing it as an anomaly detection task in the space of possible AV states concerning all the other agents and objects in the simulated world.
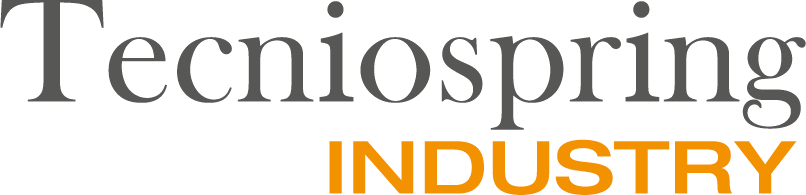

